- 1Institute for Prevention and Cancer Epidemiology, Faculty of Medicine and Medical Center, University of Freiburg, Freiburg, Germany
- 2Competence Network Preventive Medicine Baden-Württemberg, Competence Area of Personalized Prevention, Freiburg, Germany
- 3Department of Epidemiology, Fielding School of Public Health, University of California, Los Angeles, Los Angeles, CA, United States
Effective preventive strategies are urgently needed to address the rising burden of non-communicable diseases such as cardiovascular disease and cancer. To date, most prevention efforts to reduce disease incidence have primarily targeted populations using “one size fits all” public health recommendations and strategies. However, the risk for complex heterogeneous diseases is based on a multitude of clinical, genetic, and environmental factors, which translate into individual sets of component causes for every person. Recent advances in genetics and multi-omics enable the use of new technologies to stratify disease risks at an individual level fostering personalized prevention. In this article, we review the main components of personalized prevention, provide examples, and discuss both emerging opportunities and remaining challenges for its implementation. We encourage physicians, health policy makers, and public health professionals to consider and apply the key elements and examples of personalized prevention laid out in this article while overcoming challenges and potential barriers to their implementation.
Introduction
The global burden of complex heterogeneous diseases with multiple etiologies, such as cancer, diabetes mellitus type 2 (DM2), and cardiovascular diseases (CVD), is rising globally. At the same time, adverse lifestyle changes at a population level also add to risk. These trends represent a serious public health concern, and indicate a need for more effective prevention strategies. To date, most preventive strategies to reduce disease burden have targeted the general public by providing “one-size-fits-all” recommendations (1). These include most “5-a-day” to improve fruit and vegetable intake or advice to “maintain a normal body weight.” While curative medicine is increasingly embracing an individualized approach (e.g., tumor boards in oncology), preventive strategies are lagging behind. Disease risk of complex heterogeneous diseases is based on multiple genetic, genotypic, phenotypic, clinical, and environmental factors, and complex gene-environment-interactions. Recent advances in “omics” technologies have offered new opportunities to stratify individual characteristics and assess potential disease risks in more detail based on individual biological data (e.g., genetic, genomic, microbiome) (2) along with sociodemographic, clinical, and environmental factors. These technologies are likely to allow the further personalization and precision of prevention strategies. As a result of the limited success of population-based prevention strategies and the development of rapidly evolving digital technologies (3), the interest of health policy-makers and professionals in more personalized prevention strategies continues to grow. In this review, we summarize the current components that have been realized, and then we review the literature and make suggestions of additional new components how to further personalize prevention, and discuss both its potential and challenges associated with its implementation.
Status quo of prevention
Around the world, various preventive interventions are recommended and offered as part of regular health care. In the USA, for example, individuals with health insurance are eligible for annual physical check-ups, which include a standardized battery of examinations and tests including weight, blood pressure, body temperature, vaccinations, screening tests, and disease (risk) biomarkers (4). In Germany, health check-ups are covered by public health insurance once for those under 35 years of age and every 3 years for those over 35 years of age (5). Both of these include a review of medical and family history, anthropometric measurements [i.e., body mass index (BMI)], some biomarkers if indicated (blood tests or, in the case of a conspicuous family history, genetic testing) and counseling with health advice on risk factors and lifestyle recommendations (e.g., weight reduction, dietary changes).
Screening programs are usually based on age and gender, e.g., biennial breast cancer screening by mammography for women aged 50–74 years (6) and annual screening for colorectal cancer by colonoscopy from age 45 in the USA (7) and from age 50 in Germany in 10 year intervals (8). In addition to these previously implemented approaches, a variety of other possibilities for future personalization will be reviewed in the following chapter.
Key components of personalized prevention
The term personalized prevention is used throughout the review, irrespective of alternative terminologies that have accrued including precision, individualized or targeted prevention (2). We define personalized prevention as approach that incorporates information on sociodemographic, clinical, anthropometrical and behavioral factors, biomarkers, omics, and gene-environmental interactions (Figure 1), in order to indicate an individual's risk for diseases. All of this information is subsequently used to personalize preventive care.
This chapter provides an overview of these key components as understood to date, and presents exemplary fields of application for personalized prevention of cancer and cardiometabolic diseases (CMD).
Behavioral interventions
Personalized preventive behavioral interventions may be more effective than general preventive recommendations in achieving long term behavior change that might affect risk of complex diseases like DM2 and CVD (9). In this section, studies that have reviewed the effectiveness of personalized preventive counseling based on personal lifestyle data from disease prevention questionnaires are discussed and evaluated. As focus has increasingly been placed on web-based approaches, the following section will mainly deal with these techniques.
Intervention studies on the effectiveness of personalized lifestyle-based advice
Table 1 shows randomized-controlled trials (RCTs), evaluating the effect of personalized lifestyle-based prevention advice. Personalized advice was based on dietary habits (10, 13, 16) and physical activity (10, 16), CVD (11, 12, 15) or CMD risk profiles (14), behavior (i.e., diet), and clinical changes (i.e., weight reduction, cholesterol), compared with general guidelines.
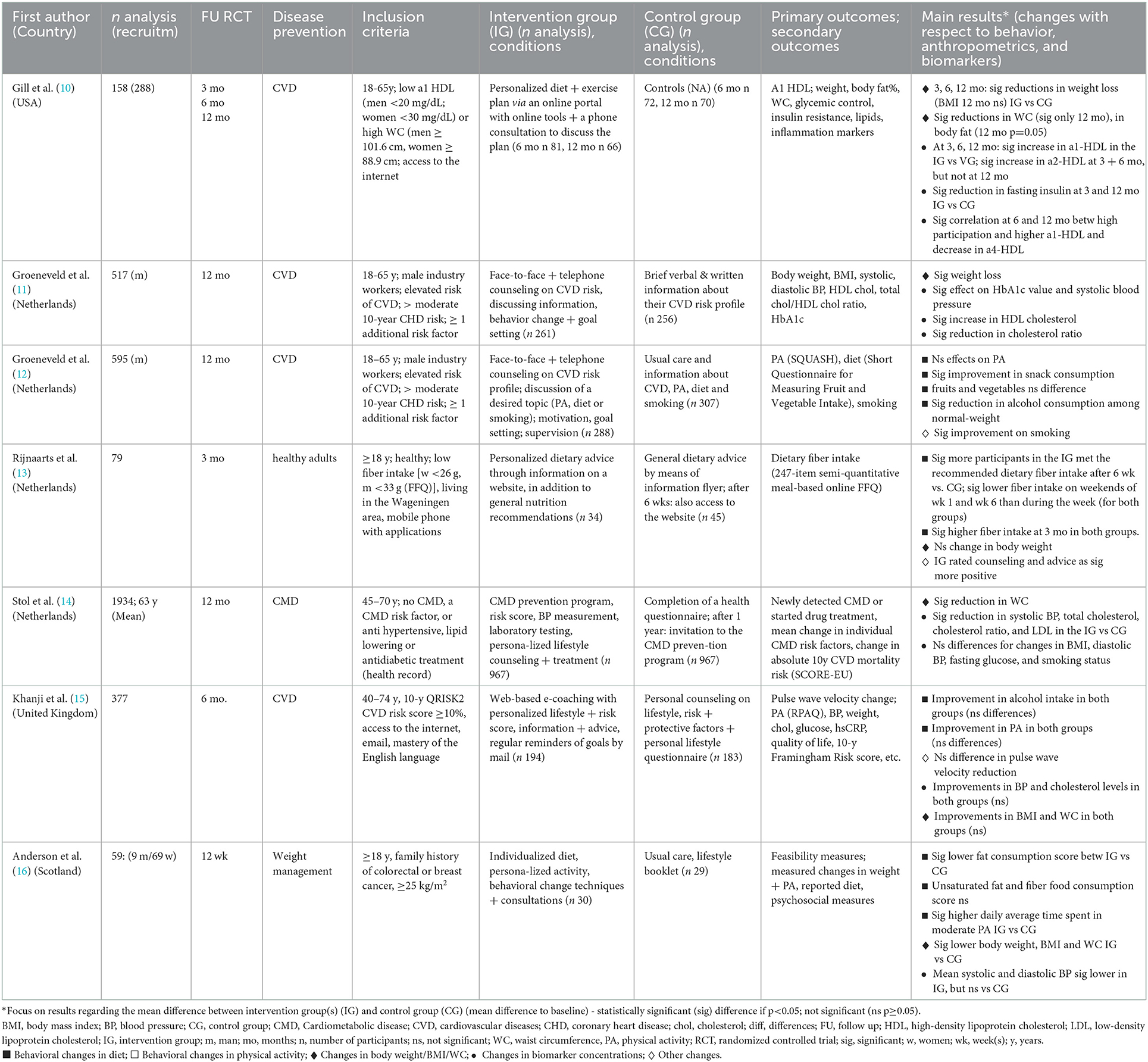
Table 1. Effects of personalized prevention based on current diet, physical activity or risk assessment on behavior, anthropometrics and biomarkers – An overview of RCTs.
With respect to dietary changes, a personalized 6-month lifestyle intervention for moderate CVD risk resulted in significant reductions in snack and fruit intake (12). Another RCT indicated that a patient group receiving personalized dietary advice on fiber intake exhibited higher adherence than a control group receiving general advice (13). A meta-analysis including 18 RCTs (1998–2011) from the USA, Netherlands and Belgium revealed higher effectiveness of e-Health personalized lifestyle interventions in increasing fruit and vegetable intake when compared to general recommendations (17).
A 12-month-intervention found significant improvement in smoking reduction only at 6 months with no effects of personalized prevention observed for physical activity (12). Furthermore, a significant reduction in alcohol consumption compared to control groups was seen only among normal-weight participants (12). In two prospective trials from the Netherlands, personalized e-Coaching lifestyle interventions led to a 20–25% relative reduction in 10-year CVD risk. However, a RCT did not reveal any effect (18).
In some studies personalized lifestyle-based interventions led to significantly greater reductions in body weight (10, 11, 16), and waist circumference (WC) (10, 14, 16), compared to the controls. However, this was not observed in other studies (13, 15). An older meta-analysis of 12 RCTs (2001–2012) targeting web-based diet and/or physical activity interventions for weight loss suggested a greater reduction in weight, BMI and WC in participants receiving web-based delivered personalized feedback compared to controls. Significant differences were apparent at both 3–6 months. These results were not found in studies with durations of 12–24 months (19).
In two studies the interventions had a significant effect on increasing high-density lipoprotein (HDL) cholesterol (10, 11). A significant reduction effect in systolic blood pressure (BP) between the groups was also observed in two studies (11, 14), but not in others (15, 16). The intervention in two RCTs led to significant reductions compared to controls in triglyceride and insulin concentrations (10) and in hemoglobin A1c levels (HbA1c) (11), a marker indicating prediabetes.
Two of the aforementioned studies (14, 15) used a stepwise prevention program for CMD that was comprised of initial individual risk assessment via validated disease risk score using a comprehensive online questionnaire (step 1), followed by additional risk profiling through laboratory tests, visiting general practitioners in case of high-risk individuals (step 2), and providing web-based individualized lifestyle advice (step 3) (10, 14). A large RCT with 967 participants with increased CMD risk and 967 controls demonstrated significant long-term decrease in WC, systolic BP, cholesterol, and LDL in the intervention compared to the control group (14). Results from the other RCT did not provide evidence of beneficial effects following personalized lifestyle counseling compared to standard of care (Table 1) (15).
Evaluation
The findings suggest that the provision of lifestyle-based risk advice might be a more useful tool for behavioral lifestyle changes compared to the use of general recommendations (1, 10–14, 16–19). However, it is not clear whether this increased motivation can be sustained over the long term (1, 11, 12, 19). Beyond that, the detection of high-risk individuals identified by disease risk scores based on sociodemographic, clinical, anthropometrical and behavioral factors, might be an effective strategy for those individuals who could most benefit from prevention strategies (14).
Biomarkers as intervention targets
Preventive approaches targeting clinical biomarker measurements are also an important pillar of personalization that already have been partly realized. Cardiometabolic biomarkers (including blood lipids, glucose, HbA1c) from laboratory testing in several combinations may provide a primary risk profile and indicate preliminary stages of CMD and their phenotypes, particularly when additional information like anthropometrics, sociodemographics, and/or familial history (Figure 1) is considered (3, 20, 21).
Improving clinical biomarkers can be achieved by various means including weight loss, physical activity, and nutritional adjustments. Nowadays, a steady expansion of the product range and marketing of nutritional supplements means that more and more consumers are taking supplements, regardless of whether or not they actually exhibit a deficient or insufficient supply of minerals, vitamins and micronutrients. A targeted supplementation of food supplements tailored to the individual deficiency should occur only when based on laboratory diagnostics.
Moreover, there is increasing evidence that the human gut microbiome plays a crucial role in immune function, human health, and disease. There is evidence to suggest that stratification of individuals according to two microbial enterotypes (either Prevotella or Bacteroides dominance) may be valuable in predicting responses to diets for obesity management (22). Furthermore, the influence of the gut microbiota on human health may be in part mediated by the ability to metabolize dietary compounds into new bacterial metabolites that may impact disease risk (23). For example, short chain-fatty acids (SCFA) produced by gut bacteria may provide a mediating link between dietary fiber intake and metabolic diseases (24).
Personalized nutrition guidance that involves biomarkers identified by various omics technologies, self-reported questionnaire-based dietary assessment tools, and useful tools to improve real-time assessment of dietary intake and feedback provision (e.g., by wearable devices and mobile apps), may have the potential for more effective glycemic control and prevention of DM2. A machine-learning algorithm that integrates particular dietary habits (using a smartphone-adjusted website), blood parameters, gut microbiota and continuous glucose monitoring was effective to predict postprandial glycemic responses to real-life meals more accurately than general dietary advice (25).
In addition, metabotyping is a new concept in which individuals are clustered into metabolic phenotypes based on clinical and biochemical parameters, anthropometric measures, metabolomics and metagenomics data. This concept, which aims to tailor diets that fit each metabotype specifically, has been suggested as a nutritional strategy, for example in the context of CMD prevention. However, health economics studies are still lacking (23).
Evaluation
As part of ongoing technological progress including molecular diagnostics, more and more biomarkers are likely to be discovered. The combination of biomarkers with sociodemographic, anthropometric, clinical and behavioral factors, and omics data can help with the identification of individuals at high risk and the detection of even pre-symptomatic chronic diseases.
Genetics, genomics and epigenetics
Interest in identifying individual genetic biomarkers as a strategy to help achieve a high degree of personalization in prevention is currently increasing. One approach is the analysis of monogenetic genes to identify increased risks for developing specific diseases. An examination of genes for coding variations likely to make individuals susceptible to breast cancer revealed an association between breast cancer risk and protein-shortening variants of these genes in ATM, BRCA1, BRCA2, Check2, and PALB2 (26).
Genome-wide association studies (GWAS) assess genetic variants across the genomes of a large number of individuals to identify genotype–phenotype associations using the technologies of whole genome sequencing and the determination of single-nucleotide polymorphisms (SNPs). Furthermore, GWAS enable the calculation of polygenic risk scores (PRS) as a measurement of risk summed over multiple risk alleles (27). In one study, a PRS for the prediction of estrogen receptor-characteristic breast cancer was developed based on 69 studies and a large data set of GWAS. Here, the optimal PRS was able to significantly predict the risk of developing breast cancer and consisted of 313 SNPs (28).
GWAS studies investigating genetic variants associated with obesity and weight loss resistance have identified several potentially relevant variants. These include the obesity predisposing FTO (fat mass and obesity associated gene) (29) and MC4R (melanocortin 4 receptor) genes related to food intake control (e.g., appetite, eating behavior) and energy homeostasis regulation (30).
However, a limitation of GWAS is the missing impact of the environment and identifying gene-environment interactions, which are both assumed to have a high influence on the individual disease risk (27, 31).
The DNA cannot be changed directly, but the function of individual genes can be influenced by epigenetic mechanisms. Epigenetics is one section of genetics which describes the variations in gene expression without a change in the DNA sequence itself (32). One main carrier of epigenetic information is DNA-methylation (33). Epigenetic alteration can cause pathologies, including cancer (31). Epigenome-wide association studies (EWAS) focus on the influence of environmental factors on the underlying mechanisms related to the development and progression of diseases (34).
Evaluation
Genetic and genomic testing seems promising for people having a family history of genetic conditions. The implementation of epigenetics has an even bigger potential for personalization due to its modifiability and the possibility of adaptations through environmental interactions.
Gene-environment interaction
Gene and environment interactions (GxE) determine the responses of individuals to environmental variation based on their (epi)genetic profile (31). Those variations can include diet and lifestyle factors.
Obesity represents a major risk factor for CMD like CVD and DM2, all of which exhibit complex interplay mechanisms of genetic and environmental risk factors (30). GxE studies have revealed evidence for decreased risk of some genetic predispositions (for example toward obesity) through the interaction between protective nutritional factors and higher levels of physical activity (30, 35, 36).
Nutrigenetics studies how genes affect nutrient metabolism (37). Large prospective studies found that increased intake of both sugar-sweetened beverages and fried food amplified the association of an obesity-related 32-SNP genetic risk score (GRS) with BMI (35). Dietary fat intake modified the association between the FTO genotype and changes in insulin sensitivity and obesity. FTO carriers exhibited a greater reduction in weight and fat distribution in response to a high-protein diet (36). While some studies observed significant interactions influencing BMI between the FTO variant and higher dietary intake of calories, proteins, saturated fat, carbohydrates and salt, one large study with 177,300 adults did not find significant interactions (35). The results of a case-control study including 7,052 high cardiovascular risk subjects suggested that a high adherence to the Mediterranean Diet counteracts the genetic predisposition toward DM2 related to FTO and MC4R (36). Furthermore, a meta-analysis revealed that higher levels of physical activity had a significantly attenuating effect on an obesity-associated 12-SNPs GRS in the American but not in the European cohorts (35).
Intervention studies on the effectiveness of phenotype- or genotype-based lifestyle advice
Table 2 summarizes key RCTs, published between 2013 and 2021, that assess the effectiveness of the provision of phenotype or genotype-based lifestyle advice on changes in anthropometrics, behavior, clinical results, and psychological factors.
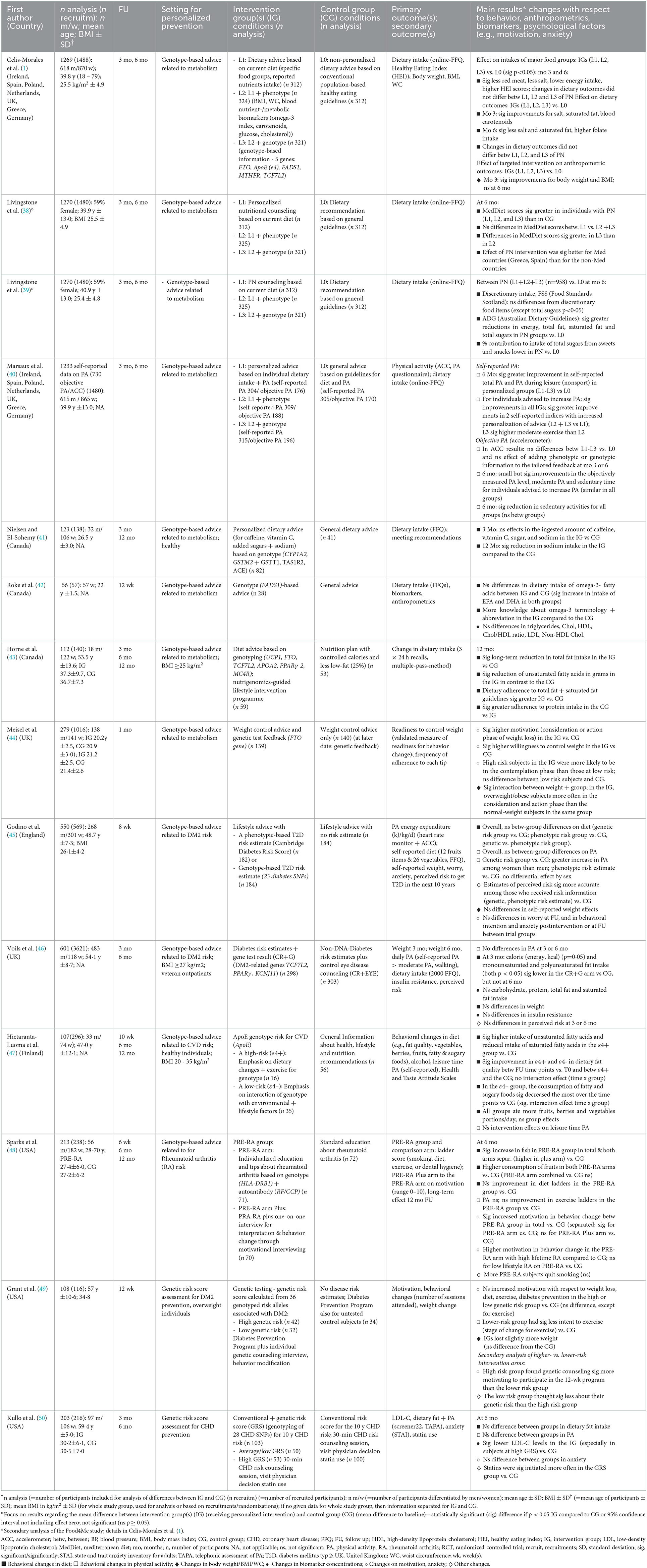
Table 2. Effects of personalized prevention based on current diet, phenotype and/or genotype on behavior, anthropometrics and biomarkers – An overview of RCTs.
To date, the Food4me study (1) is the largest and most complex RCT, including 1,269 adults from seven European countries. This study assesses the effectiveness of three different web-based types of personalized nutrition advice on changing eating patterns (compared to generalized dietary advice). The results of the study's 6-month intervention period demonstrated that personalized advice based on the individual's dietary intake and weight was more effective in motivating participants to make healthy changes to their usual diet than the conventional dietary recommendations received by the control group. However, no evidence of enhanced effectiveness from the use of more in-depth personalization in terms of phenotypic and genotypic information was observed (1, 39). Secondary analyses pointed to significantly lower sugar intake (39) and greater Mediterranean Diet scores (MDS) (38). Interestingly, differences in MDS were significantly greater in participants receiving dietary advice based on current diet, phenotype and genotype than in participants providing advice based on current diet plus phenotype only (38).
Further RCTs exhibited significant reductions in sodium intake (41), and total fat and unsaturated fatty acids (43), in the metabolic genotype-based dietary advice group compared to controls. In another RCT, no significant differences in dietary intake of omega-3-fatty acids and biomarkers (e.g., triglyceride, cholesterol) between the groups were observed (42). Following the provision of genotype-based lifestyle advice related to disease risk, RCTs found significant improvements on fat intake (46, 47), reductions in sugar intake (47), and an increase in fruits and fish consumption (48) compared to the controls. However, another RCT observed no differences in any of the food groups resulting from personalized nutrition advice (45).
Additionally, data from the Food4me study did not find evidence for higher effectiveness of personalized web-based advice on increasing physical activity (when measured via accelerometer) compared to the use of general guidelines (40). In contrast, self-reported physical activity significantly increased to a greater extent with higher degrees of personalized advice (40). Further RCTs revealed no significant effects of genotype-based lifestyle advice on physical activity [based on self-reports (46–48), or accelerometer (45)] compared to the controls.
With respect to weight reduction, in the Food4me study, significant effects on weight loss made by participants receiving any type of personalized nutrition were significant at three, but not at 6 months. These changes did not differ based the type of personalization (1). In contrast, no significant effects on weight between the genotype- (45, 46) or phenotypic-based risk communication group (45) compared to the control groups were observed in other studies (45, 46).
A revised version of a Cochrane review and meta-analysis from 2016 (including 18 RCTs published until 2015) did not reveal significant effects of communicating DNA based risk estimates on diet, physical activity, smoking cessation, alcohol use, or behavioral support programs (51). A cohort study including 2,037 participants, who received genomic data with provided estimates of lifetime risk of developing 18 common health conditions, did not indicate significant changes on dietary intake or exercise following delivery of genomic profiling (52). Furthermore, surveys on direct-to-consumer genetic testing (DTC-GT) have suggested that disease-specific information on genetic predisposition may motivate individuals slightly more in terms of diet and physical activity (53).
The evidence regarding the effectiveness of personalized prevention in the long-term (≥6 months) during the intervention phase is not clear. While in some RCTs only one follow-up time point was recorded and analyzed [ ≤ 3 (42, 44, 45, 49) and 6 months (38, 39)], some studies also analyzed the effects over time with two [3 and 6 months (1, 40, 46, 50); 3 and 12 months (41)] or three analyzed time points (43, 47, 48) (Table 2). With regard to RCTs assessing changes in dietary behavior and weight, some studies pointed to significant improvements in at least one outcome both in the short- and long-term [total fat, unsaturated fatty acids (43); red meat, salt, energy intake, Healthy Eating Index, saturated fat (1)]. On the other hand, different RCTs indicated significant improvements only in the short-term but not in the long-term [blood carotenoids, body weight (1); fat intake (46)]. However, other studies let to significant improvements in the long-term but not in the short-term [folate intake (1); sodium intake (41)].
Impact of genetic risk communication on motivation and adverse effects
The motivational effect of personalized prevention can be directly measured by lifestyle changes and improvements in biomarkers. However, few RCTs have investigated the motivational effect of genetic- or genotype-based communication interventions on personal motivation for lifestyle changes (44, 48, 49) (Table 2). The older Cochrane meta-analysis of RCTs did not conclude that communicating DNA based risk estimates had any measurable effect on motivation or intention to change behavior (51). However, more recent RCTs (44, 48) have concluded that the provision of personalized genotype-based lifestyle advice can increase the motivation of participants to integrate changed behavior into their daily life. Furthermore, studies investigating whether or not the motivational effect of personalized prevention last beyond the intervention are still lacking. However, web-based approaches including techniques and reminders might support long-term behavior changes for CMD prevention. One current systematic review concluded that telemedicine platforms may be used for regular check-ups for preventive care of CVD (54).
With respect to possible adverse effects, two RCTs have reported that communication of genetic risk does not seem to increase anxiety (45, 50). Neither the results from the Cochrane meta-analysis nor the results from an online survey following DTC-GT indicated an impact of genetic risk communication on levels of depression (51) and anxiety (51, 52).
Evaluation
In summary, the largest study to date (1) revealed that personalized nutrition advice let to healthier diet compared with controls, despite no evidence of any additional benefit from using more in-depth personalization in terms of phenotypic and genotypic information were demonstrated. However, some smaller studies reported that the provision of genetic- or genotypic based risk advice improved dietary behavior in one or more dietary outcomes compared to general advice (38, 39, 41, 43–50). Yet, the long-term effects of web-based personalized preventive interventions are still unclear. Therefore, personal contact and guidance via face-to-face or web-based human-delivered counseling seem to be essential. The presented findings also suggest that the provision of a genetic-based risk advice may motivate healthy behavior changes, and that communication of genetic risk does not arise to predict adverse effects like anxiety.
Prospects of personalized prevention
Personalized prevention offers promising opportunities as summarized in Figure 2. Personalized prevention can help with the prediction of disease occurrence and can be implemented in ways that are targeted to an individual profile of genomic data and other biomarkers (55). In addition, personalized preventive approaches can lead to an improvement in the quality of care and quality of life of patients and furthermore to a reduction in healthcare time, effort and costs (55–57). Specific personalized preventive interventions could be exclusively provided to high-risk individuals for developing a particular disease based on risk calculations. This could therefore lead to far more cost-effective outcomes (58), particularly through the avoidance of over-diagnosis and redundant treatment (59). Nevertheless, more cost-effective calculations are needed, especially for omics-testing technologies (55). Further research about behavioral science interventions will be needed in order to ensure a sustainable integration of personalized preventive interventions and behaviors (55).
The aim of the German National Cohort is to investigate the causes of diseases through long-term monitoring, to discover and validate new biomarkers, and to develop preventive measures. Progress in biomarker research will enable continuous improvement in the early detection of chronic diseases and their precursors (60).
Implementational challenges of personalized prevention
The incorporation of personalized prevention into health practice remains limited. Healthcare systems face a variety of challenges that stand in the way of a successful realization of the full potential of this approach. One of the greatest long-term challenges is the implementation of policies. In their 2021 review, Trein and Wagner give an overview of the four most relevant policy challenges found in the literature for implementing precision health (including personalized prevention) in practice: (1) creating, maintaining and harmonizing an infrastructure for research, (2) building and fostering trust in precision health amongst citizens in general and patients in particular, (3) establishing regulatory frameworks to ensure cooperation and to avoid discrimination, and (4) integrating precision health into existing health systems (61).
Genetic testing
In order to incorporate more genetic testing into medical procedures in the future, the acceptance of these technologies by the general public will be an important factor (62). Previous systematic reviews report mixed attitudes toward genetic testing, with a higher number of studies reporting positive attitudes (62, 63). Participants perceived benefits from genetic testing, especially for prevention and treatment of diseases (63). The primary areas of public concern relate to the privacy of genetic information, with a special fear of misuse in the form of genetic discrimination (GD) (62, 63). The most researched domains that could be affected by GD are insurance and employment settings (64, 65). One negative consequence of these concerns could be an unwillingness to undergo genetic testing among individuals high at risk, which could cause increased manifestations of disease and death (64). Numerous policies, laws and strategies have been developed in different countries to prevent GD in insurance and employment settings. These include GINA (Genetic Information Nondiscrimination Act) in the USA (64), and the GenDG (Genetic Diagnostics Act) in Germany (66). All of these “explicitly proscribe discrimination based on the DNA composition of an individual's genome” (64). However, the definition of genetic information used in these policies is greatly limited and does not include new precision health biomarkers like omics-technologies. Thus, current literature identifies an urgent need for developing international standards, collaborative initiatives, and revised national policies addressing GD in the future (64, 65). The fear of GD could lead to the use of DTC-GT approaches outside the regular healthcare system as a result of consumers hoping to bypass potential GD (66). The implementation of DTC-GT in healthcare may seem promising, but some concerns remain. These include worry that new technologies may not always be covered by anti-discrimination or data protection laws, and concerns of lacking medical communication of the results. Genetic counseling with genetic health professionals should always take place in order to adequately discuss genetic results.
Education
For a successful implementation of personalized prevention in health care practice, a better understanding of personalized concepts and technologies among healthcare providers is crucial. The demand for appropriately trained health professionals is currently growing. Therefore, the continued development of educational programs and training in omics sciences, personalized communication skills and personalized prevention concepts in general will be of key importance. It will be both necessary to define core competencies that can be integrated into the curricula of health care professional training programs and to develop national policies and regulatory frameworks that ensure the integration of adequate personalized preventive practice (56).
Health literacy and an understanding of the benefits and limits of personalized preventive technologies like omics-testing is needed for citizen empowerment and engagement in both contributing to research and adopting new behaviors in everyday life (56, 57, 67). This will only be possible if educational, ethical, socioeconomic and cultural obstacles are correctly addressed and managed (55). These topics must be addressed cross-sectionally and therefore, perspectives from experts in different health care disciplines must be integrated (2, 55).
Health disparities
Guaranteed access to healthcare for all is a crucial goal that will require focused and sustained effort. The elimination of health disparities will require system changes that promote health equities and access to high quality care for all (68). Social, ethnic, economic and regional disparities are still not appropriately addressed (67).
A lack of ethnic diversity in the data creates a disturbing potential for the exacerbation of already existing health disparities. For future research, it is therefore essential that study samples include diverse patient populations (different ethnicities and socially disadvantaged populations) to ensure that scientific advances benefit all populations (55, 68, 69). Besides biomarkers, data regarding environmental, socioeconomic, sociocultural, and behavioral factors should be collected for a holistic understanding of influences on the development of diseases (68). Furthermore, personalized telemedical approaches have the potential to reach populations that are otherwise difficult to reach and make it possible to collect more diverse digital biomarkers (59).
Few reviews have summarized strategies and recommendations for the implementation of personalized medicine into preventative care. The recommendations most mentioned in the literature include: (1) develop education programs for personalized prevention and the building of community awareness, (2) empower and engage patients, (3) develop infrastructure and information management systems, (4) value recognition for precision health, and (5) ensure access to care (67, 70). Table 3 presents different challenges and barriers for a successful implementation of personalized prevention in healthcare practice, along with potential strategies to overcome them.
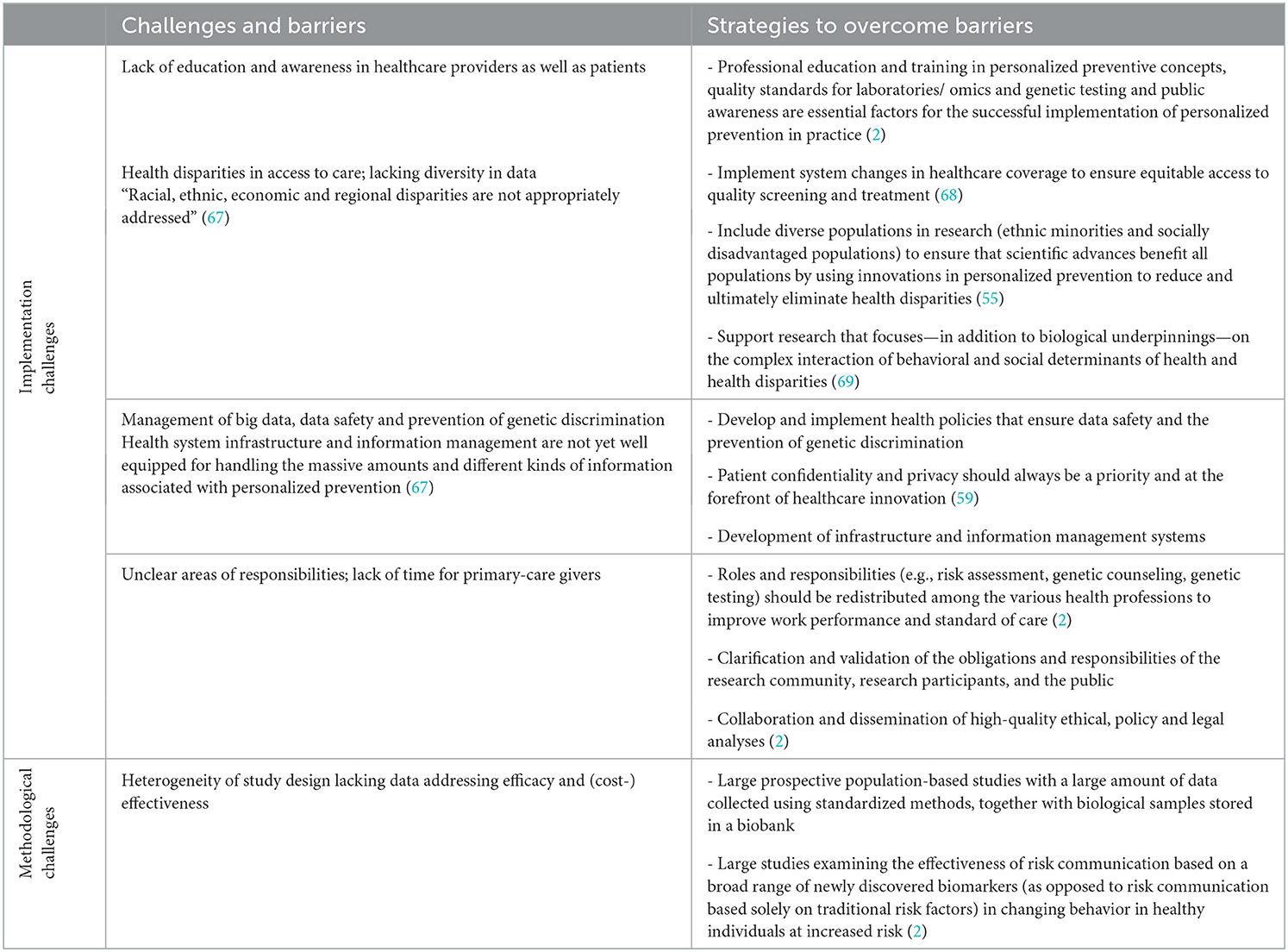
Table 3. Challenges and barriers for a successful implementation of personalized prevention in healthcare practice and strategies to overcome those.
Implementation and resistance
Current prevention strategies and recommendations are largely based on the results from large population-based studies and target shifts in the entire population. Public health prevention is often viewed as an altruistic principle which values the benefit of the population above that of the individual (e.g., vaccination). Such population-strategies of prevention are in contrast with a personalized prevention approach. Thus, the implementation of personalized prevention strategies dependent at least in part upon the public health infrastructure (71).
Future perspectives
Personalized prevention demonstrates great potential to increase the effectiveness of preventive interventions. However, achieving the successful integration of personalized prevention into everyday health care will require changes in health care systems. The pursuit of this goal will require all stakeholders to work to enable the implementation of personalized prevention. Along with improving the training of health practitioners, policies, regulations, and companies will also have to adapt. Some of the required changes will require long term implementation. These include structural changes in the healthcare system and the development of policies and legal foundations to address issues like health disparities and genetic discrimination. In terms of changes that may be achieved in the short term, we see great potential in advancing the education of health professions in omics sciences and enhancing citizen awareness and engagement. For the clinical setting, we recommend further development of personalized prevention techniques using predictive biomarkers as well as telemedical approaches in primary care institutions. Personalized prevention offers a variety of promising opportunities. Therefore, we encourage physicians, health policy makers, and public health professionals to consider and apply the key elements of personalized prevention into practice.
Author contributions
KM obtained funding and critically reviewed the manuscript. SJ, CN, and KM conceptualized the review, contributed to manuscript revision, and have responsibility for final content. SJ and CN wrote the manuscript and did the literature research, study selection, and interpretation. SJ created the figures. All authors read and approved the final manuscript.
Funding
This review was funded by the Ministry of Science, Research and the Arts of Baden-Württemberg (BW) AZ 34-04HV.MED(22)/18-1; Funding Program Cooperation Network University Medicine BW-Serial No. 66 (Competence Network Preventive Medicine BW, Share MFFR).
Conflict of interest
The authors declare that the research was conducted in the absence of any commercial or financial relationships that could be construed as a potential conflict of interest.
The reviewer HP declared a shared affiliation with the author(s) KM to the handling editor at the time of review.
Publisher's note
All claims expressed in this article are solely those of the authors and do not necessarily represent those of their affiliated organizations, or those of the publisher, the editors and the reviewers. Any product that may be evaluated in this article, or claim that may be made by its manufacturer, is not guaranteed or endorsed by the publisher.
References
1. Celis-Morales C, Livingstone KM, Marsaux CFM, Macready AL, Fallaize R, O'Donovan CB, et al. Effect of personalized nutrition on health-related behaviour change: evidence from the Food4me European randomized controlled trial. Int J Epidemiol. (2017) 46:578–88. doi: 10.1093/ije/dyw186
2. Boccia S, Pastorino R, Ricciardi W, Ádány R, Barnhoorn F, Boffetta P, et al. How to integrate personalized medicine into prevention? Recommendations from the personalized prevention of chronic diseases (PRECeDI) consortium. Public Health Genom. (2019) 22:208–14. doi: 10.1159/000504652
3. Wang DD, Hu FB. Precision nutrition for prevention and management of type 2 diabetes. Lancet Diabetes Endocrinol. (2018) 6:416–26. doi: 10.1016/S2213-8587(18)30037-8
4. U.S. Department of Health and Human Services. Preventive Care Benefits for Adults. New York, NY: U.S. Department of Health and Human Services (2022). Available online at: https://www.healthcare.gov/preventive-care-adults/ (accessed June 2, 2022)
5. Gemeinsamer Bundesausschuss. Richtlinie des Gemeinsamen Bundesausschusses über die Gesundheitsuntersuchungen zu Früherkennung von Krankheiten. Berlin: Gemeinsamer Bundesausschuss (2019). Available online at: https://www.g-ba.de/downloads/62-492-2605/oKFE-RL-2021-07-01-iK-2022-01-01.pdf (accessed March 24, 2022).
6. Siu AL, US Preventive Services Task Force. Screening for breast cancer: US preventive services task force recommendation statement. Ann Intern Med. (2016) 164:279–96. doi: 10.7326/M15-2886
7. Preventive Services, Task US, Barry MJ, Mangione CM, Cabana M, Caughey AB, et al. Screening for colorectal cancer: US preventive services task force recommendation statement. JAMA. (2021) 325:1965–77. doi: 10.1001/jama.2021.6238
8. Gemeinsamen Bundesausschusses. Richtlinie des Gemeinsamen Bundesausschusses für organisierte Krebsfrüherkennungsprogramme. Berlin: Gemeinsamen Bundesausschusses (2018). Available online at: https://www.g-ba.de/downloads/62-492-2605/oKFE-RL-2021-07-01-iK-2022-01-01.pdf (accessed March 24, 2022)
9. Ordovas JM, Ferguson LR, Tai ES, Mathers JC. Personalised nutrition and health. BMJ. (2018) 2018:361. doi: 10.1136/bmj.k2173
10. Gill R, Superko HR, McCarthy MM, Jack K, Jones B, Ghosh D, et al. Cardiovascular risk factor reduction in first responders resulting from an individualized lifestyle and blood test program: a randomized controlled trial. J Occup Environ Med. (2019) 61:183–9. doi: 10.1097/JOM.0000000000001490
11. Groeneveld IF, Proper KI, van der Beek AJ, van Mechelen W. Sustained body weight reduction by an individual-based lifestyle intervention for workers in the construction industry at risk for cardiovascular disease: results of a randomized controlled trial. Prevent Med. (2010) 51:240–6. doi: 10.1016/j.ypmed.2010.07.021
12. Groeneveld IF, Proper KI, van der Beek AJ, Hildebrandt VH, van Mechelen W. Short and long term effects of a lifestyle intervention for construction workers at risk for cardiovascular disease: a randomized controlled trial. BMC Public Health. (2011) 11:836. doi: 10.1186/1471-2458-11-836
13. Rijnaarts I, de Roos NM, Wang T, Zoetendal EG, Top J, Timmer M, et al. Increasing dietary fibre intake in healthy adults using personalised dietary advice compared with general advice: a single-blind randomised controlled trial. Public Health Nutr. (2021) 24:1117–28. doi: 10.1017/S1368980020002980
14. Stol DM, Badenbroek IF, Hollander M, Nielen MMJ, Kraaijenhagen RA, Schellevis FG, et al. Effectiveness of a stepwise cardiometabolic disease prevention program: results of a randomized controlled trial in primary care. Prevent Med. (2020) 132:105984. doi: 10.1016/j.ypmed.2020.105984
15. Khanji MY, Balawon A, Boubertakh R, Hofstra L, Narula J, Hunink M, et al. Personalized E-coaching in cardiovascular risk reduction: a randomized controlled trial. Ann Global Health. (2019) 85:107. doi: 10.5334/aogh.2496
16. Anderson AS, Dunlop J, Gallant S, Macleod M, Miedzybrodzka Z, Mutrie N, et al. Feasibility study to assess the impact of a lifestyle intervention (“LivingWELL”) in people having an assessment of their family history of colorectal or breast cancer. BMJ Open. (2018) 8:e019410. doi: 10.1136/bmjopen-2017-019410
17. Celis-Morales C, Lara J, Mathers JC. Personalising nutritional guidance for more effective behaviour change. Proc Nutr Soc. (2015) 74:130–8. doi: 10.1017/S0029665114001633
18. Yousuf H, Reintjens R, Slipszenko E, Blok S, Somsen GA, Tulevski II, et al. Effectiveness of web-based personalised e-Coaching lifestyle interventions. Neth Heart J. (2019) 27:24–9. doi: 10.1007/s12471-018-1200-7
19. Sherrington A, Newham JJ, Bell R, Adamson A, McColl E, Araujo-Soares V. Systematic review and meta-analysis of internet-delivered interventions providing personalized feedback for weight loss in overweight and obese adults: internet-based weight loss interventions: impact of feedback. Obes Rev. (2016) 17:541–51. doi: 10.1111/obr.12396
20. Stefan N, Fritsche A, Schick F, Häring H-U. Phenotypes of prediabetes and stratification of cardiometabolic risk. Lancet Diabet Endocrinol. (2016) 4:789–98. doi: 10.1016/S2213-8587(16)00082-6
21. Piché M-E, Tchernof A, Després J-P. Obesity phenotypes, diabetes, and cardiovascular diseases. Circ Res. (2020) 126:1477–500. doi: 10.1161/CIRCRESAHA.120.316101
22. Christensen L, Roager HM, Astrup A, Hjorth MF. Microbial enterotypes in personalized nutrition and obesity management. Am J Clin Nutr. (2018) 108:645–51. doi: 10.1093/ajcn/nqy175
23. Palmnäs M, Brunius C, Shi L, Rostgaard-Hansen A, Torres NE, González-Domínguez R, et al. Perspective: metabotyping—a potential personalized nutrition strategy for precision prevention of cardiometabolic disease. Adv Nutr. (2020) 11:524–32. doi: 10.1093/advances/nmz121
24. Sanna S, van Zuydam NR, Mahajan A, Kurilshikov A, Vich Vila A, Võsa U, et al. Causal relationships among the gut microbiome, short-chain fatty acids and metabolic diseases. Nat Genet. (2019) 51:600–5. doi: 10.1038/s41588-019-0350-x
25. Zeevi D, Korem T, Zmora N, Israeli D, Rothschild D, Weinberger A, et al. Personalized nutrition by prediction of glycemic responses. Cell. (2015) 163:1079–94. doi: 10.1016/j.cell.2015.11.001
26. Breast Cancer Association Consortium, Dorling L, Carvalho S, Allen J, González-Neira A, Luccarini C, et al. Breast cancer risk genes—association analysis in more than 113,000 women. N Engl J Med. (2021) 384:428–39. doi: 10.1186/s13073-022-01052-8
27. Tam V, Patel N, Turcotte M, Bossé Y, Paré G, Meyre D. Benefits and limitations of genome-wide association studies. Nat Rev Genet. (2019) 20:467–84. doi: 10.1038/s41576-019-0127-1
28. Lee A, Mavaddat N, Wilcox AN, Cunningham AP, Carver T, Hartley S, et al. BOADICEA: a comprehensive breast cancer risk prediction model incorporating genetic and nongenetic risk factors. Genet Med. (2019) 21:1708–18. doi: 10.1038/s41436-018-0406-9
29. Ferguson LR, De Caterina R, Görman U, Allayee H, Kohlmeier M, Prasad C, et al. Guide and position of the international society of nutrigenetics/nutrigenomics on personalised nutrition: part 1—fields of precision nutrition. Lifestyle Genom. (2016) 9:12–27. doi: 10.1159/000445350
30. Barrea L, Annunziata G, Bordoni L, Muscogiuri G, Colao A, Savastano S, et al. Nutrigenetics—personalized nutrition in obesity and cardiovascular diseases. Int J Obes Supp. (2020) 10:1–13. doi: 10.1038/s41367-020-0014-4
31. Feinberg AP. The key role of epigenetics in human disease prevention and mitigation. N Engl J Med. (2018) 378:1323–34. doi: 10.1056/NEJMra1402513
32. Egger G, Liang G, Aparicio A, Jones PA. Epigenetics in human disease and prospects for epigenetic therapy. Nature. (2004) 429:457–63. doi: 10.1038/nature02625
33. Cavalli G, Heard E. Advances in epigenetics link genetics to the environment and disease. Nature. (2019) 571:489–99. doi: 10.1038/s41586-019-1411-0
34. Wei S, Tao J, Xu J, Chen X, Wang Z, Zhang N, et al. Ten years of EWAS. Adv Sci. (2021) 8:2100727. doi: 10.1002/advs.202100727
35. Goodarzi MO. Genetics of obesity: what genetic association studies have taught us about the biology of obesity and its complications. Lancet Diabet Endocrinol. (2018) 6:223–36. doi: 10.1016/S2213-8587(17)30200-0
36. Ramos-Lopez O, Milagro FI, Allayee H, Chmurzynska A, Choi MS, Curi R, et al. Guide for current nutrigenetic, nutrigenomic, and nutriepigenetic approaches for precision nutrition involving the prevention and management of chronic diseases associated with obesity. J Nutrigenet Nutrigenom. (2017) 10:43–62. doi: 10.1159/000477729
37. Sampson S. Nutrigenomics and nutrigenetics in functional foods and personal nutrition. J Nutr Edu Behav. (2015) 47:574.e3. doi: 10.1016/j.jneb.2015.06.005
38. Livingstone KM, Celis-Morales C, Navas-Carretero S, San-Cristobal R, Macready AL, Fallaize R, et al. Effect of an Internet-based, personalized nutrition randomized trial on dietary changes associated with the Mediterranean diet: the Food4Me Study. Am J Clin Nutr. (2016) 104:288–97. doi: 10.3945/ajcn.115.129049
39. Livingstone KM, Celis-Morales C, Navas-Carretero S, San-Cristobal R, Forster H, Woolhead C, et al. Personalised nutrition advice reduces intake of discretionary foods and beverages: findings from the Food4Me randomised controlled trial. Int J Behav Nutr Phys Act. (2021) 18:70. doi: 10.1186/s12966-021-01136-5
40. Marsaux CF, Celis-Morales C, Fallaize R, Macready AL, Kolossa S, Woolhead C, et al. Effects of a web-based personalized intervention on physical activity in european adults: a randomized controlled trial. J Med Internet Res. (2015) 17:e231. doi: 10.2196/jmir.4660
41. Nielsen DE, El-Sohemy A. Disclosure of genetic information and change in dietary intake: a randomized controlled trial. PLoS ONE. (2014) 9:e112665. doi: 10.1371/journal.pone.0112665
42. Roke K, Walton K, Klingel S, Harnett A, Subedi S, Haines J, et al. Evaluating changes in omega-3 fatty acid intake after receiving personal FADS1 genetic information: a randomized nutrigenetic intervention. Nutrients. (2017) 9:240. doi: 10.3390/nu9030240
43. Horne J, Gilliland J, O'Connor C, Seabrook J, Madill J. Enhanced long-term dietary change and adherence in a nutrigenomics-guided lifestyle intervention compared to a population-based (GLB/DPP) lifestyle intervention for weight management: results from the NOW randomised controlled trial. BMJNPH. (2020) 3:49–59. doi: 10.1136/bmjnph-2020-000073
44. Meisel SF, Beeken RJ, van Jaarsveld CHM, Wardle J. Genetic susceptibility testing and readiness to control weight: results from a randomized controlled trial—effects of genetic testing for weight control. Obesity. (2015) 23:305–12. doi: 10.1002/oby.20958
45. Godino JG, van Sluijs EMF, Marteau TM, Sutton S, Sharp SJ, Griffin SJ. Lifestyle advice combined with personalized estimates of genetic or phenotypic risk of type 2 diabetes, and objectively measured physical activity: a randomized controlled trial. PLoS Med. (2016) 13:e1002185. doi: 10.1371/journal.pmed.1002185
46. Voils CI, Coffman CJ, Grubber JM, Edelman D, Sadeghpour A, Maciejewski ML, et al. Does type 2 diabetes genetic testing and counseling reduce modifiable risk factors? A randomized controlled trial of veterans. J Gen Intern Med. (2015) 30:1591–8. doi: 10.1007/s11606-015-3315-5
47. Hietaranta-Luoma H-L, Tahvonen R, Iso-Touru T, Puolijoki H, Hopia A. An intervention study of individual, apoE genotype-based dietary and physical-activity advice: impact on health behavior. J Nutrigenet Nutrigenom. (2014) 7:161–74. doi: 10.1159/000371743
48. Sparks JA, Iversen MD Yu Z, Triedman NA, Prado MG, Miller Kroouze R, et al. Disclosure of personalized rheumatoid arthritis risk using genetics, biomarkers, and lifestyle factors to motivate health behavior improvements: a randomized controlled trial. Arthritis Care Res. (2018) 70:823–33. doi: 10.1002/acr.23411
49. Grant RW, O'Brien KE, Waxler JL, Vassy JL, Delahanty LM, Bissett LG, et al. Personalized genetic risk counseling to motivate diabetes prevention. Diabetes Care. (2013) 36:13–9. doi: 10.2337/dc12-0884
50. Kullo IJ, Jouni H, Austin EE, Brown S-A, Kruisselbrink TM, Isseh IN, et al. Incorporating a genetic risk score into coronary heart disease risk estimates: effect on low-density lipoprotein cholesterol levels (the MI-GENES Clinical Trial). Circulation. (2016) 133:1181–8. doi: 10.1161/CIRCULATIONAHA.115.020109
51. Hollands GJ, French DP, Griffin SJ, Prevost AT, Sutton S, King S, et al. The impact of communicating genetic risks of disease on risk-reducing health behaviour: systematic review with meta-analysis. BMJ. (2016) 2016:i1102. doi: 10.1136/bmj.i1102
52. O'Donovan CB, Walsh MC, Gibney MJ, Brennan L, Gibney ER. Knowing your genes: does this impact behaviour change? Proc Nutr Soc. (2017) 76:182–91. doi: 10.1017/S0029665116002949
53. Guasch-Ferré M, Dashti HS, Merino J. Nutritional genomics and direct-to-consumer genetic testing: an overview. Adv Nutr. (2018) 9:128–35. doi: 10.1093/advances/nmy001
54. Battineni G, Sagaro GG, Chintalapudi N, Amenta F. The benefits of telemedicine in personalized prevention of cardiovascular diseases (CVD): a systematic review. JPM. (2021) 11:658. doi: 10.3390/jpm11070658
55. Pastorino R, Loreti C, Giovannini S, Ricciardi W, Padua L, Boccia S. Challenges of prevention for a sustainable personalized medicine. JPM. (2021) 11:311. doi: 10.3390/jpm11040311
56. Traversi D, Pulliero A, Izzotti A, Franchitti E, Iacoviello L, Gianfagna F, et al. Precision medicine and public health: new challenges for effective and sustainable health. JPM. (2021) 11:135. doi: 10.3390/jpm11020135
57. Strianese O, Rizzo F, Ciccarelli M, Galasso G, D'Agostino Y, Salvati A, et al. Precision and personalized medicine: how genomic approach improves the management of cardiovascular and neurodegenerative disease. Genes. (2020) 11:747. doi: 10.3390/genes11070747
58. Cohen JT, Neumann PJ, Weinstein MC. Does preventive care save money? Health economics and the presidential candidates. N Engl J Med. (2008) 358:661–3. doi: 10.1056/NEJMp0708558
59. Au R, Ritchie M, Hardy S, Ang TFA, Lin H. Aging well: using precision to drive down costs and increase health quality. Adv Geriatr Med Res. (2019) 1:386. doi: 10.20900/agmr20190003
60. German National Cohort (GNC) Consortium. The German National Cohort: aims, study design and organization. Eur J Epidemiol. (2014) 29:371–82. doi: 10.1007/s10654-014-9890-7
61. Trein P, Wagner J. Governing personalized health: a scoping review. Front Genet. (2021) 12:650504. doi: 10.3389/fgene.2021.650504
62. LePoire E, Basu B, Walker L, Bowen DJ. What do people think about genetics? A systematic review. J Community Genet. (2019) 10:171–87. doi: 10.1007/s12687-018-0394-0
63. Calabrò GE, Sassano M, Tognetto A, Boccia S. Citizens' attitudes, knowledge, and educational needs in the field of omics sciences: a systematic literature review. Front Genet. (2020) 11:570649. doi: 10.3389/fgene.2020.570649
64. Joly Y, Dupras C, Pinkesz M, Tovino SA, Rothstein MA. Looking beyond GINA: policy approaches to address genetic discrimination. Annu Rev Genom Hum Genet. (2020) 21:491–507. doi: 10.1146/annurev-genom-111119-011436
65. Chapman CR, Mehta KS, Parent B, Caplan AL. Genetic discrimination: emerging ethical challenges in the context of advancing technology. J Law Biosci. (2020) 7:lsz016. doi: 10.1093/jlb/lsz016
66. Borry P, van Hellemondt RE, Sprumont D, Jales CFD, Rial-Sebbag E, Spranger TM, et al. Legislation on direct-to-consumer genetic testing in seven European countries. Eur J Hum Genet. (2012) 20:715–21. doi: 10.1038/ejhg.2011.278
67. Pritchard DE, Moeckel F, Villa MS, Housman LT, McCarty CA, McLeod HL. Strategies for integrating personalized medicine into healthcare practice. Person Med. (2017) 14:141–52. doi: 10.2217/pme-2016-0064
68. Zavala VA, Bracci PM, Carethers JM, Carvajal-Carmona L, Coggins NB, Cruz-Correa MR, et al. Cancer health disparities in racial/ethnic minorities in the United States. Br J Cancer. (2021) 124:315–32. doi: 10.1038/s41416-020-01038-6
69. Menon U, Ashing K, Chang MW, Christy SM, Friberg-Felsted K, Rivas VG, et al. Application of the ConNECT framework to precision health and health disparities. Nurs Res. (2019) 68:99–109. doi: 10.1097/NNR.0000000000000329
70. Qoronfleh MW, Chouchane L, Mifsud B, Al Emadi M, Ismail S, THE FUTURE OF MEDICINE. healthcare innovation through precision medicine: policy case study of Qatar. Life Sci Soc Policy. (2020) 16:12. doi: 10.1186/s40504-020-00107-1
Keywords: personalized prevention, precision health, nutrigenetics, nutrigenomics, predictive biomarkers, precision health policies, genetic discrimination
Citation: Jaskulski S, Nuszbaum C and Michels KB (2023) Components, prospects and challenges of personalized prevention. Front. Public Health 11:1075076. doi: 10.3389/fpubh.2023.1075076
Received: 20 October 2022; Accepted: 09 January 2023;
Published: 16 February 2023.
Edited by:
Alexey N. Meshkov, National Research Center for Preventive Medicine, RussiaReviewed by:
Hannah Lui Park, University of California, Irvine, United StatesJohn Groopman, Johns Hopkins Medicine, United States
Copyright © 2023 Jaskulski, Nuszbaum and Michels. This is an open-access article distributed under the terms of the Creative Commons Attribution License (CC BY). The use, distribution or reproduction in other forums is permitted, provided the original author(s) and the copyright owner(s) are credited and that the original publication in this journal is cited, in accordance with accepted academic practice. No use, distribution or reproduction is permitted which does not comply with these terms.
*Correspondence: Karin B. Michels, tumorepidemiologie@uniklinik-freiburg.de